A New AI Series on CPO Rising
The power of artificial intelligence (AI) is undeniable. Its impact and influence are revolutionizing the Procurement automation market. According to research from Ardent Partners, AI will soon be ubiquitous in this sector, offering unparalleled efficiencies and capabilities. The transformative possibilities of AI will drive innovations in source to pay, eSourcing, risk management, and overall supply chain management, streamlining enterprise operations and improving business decision-making.
In view of these advancements, Ardent Partners is excited to announce a special “AI in Action” interview series. We invite your company to participate in this series and contribute to the thought leadership around AI by having a product or strategy executive respond to high-level questions from Andrew Bartolini, our Founder and Chief Research Officer for Ardent Partners.
Today’s AI in Action profile features a conversation with Yuan Tung, Chief Technology Officer and Co-founder of ORO Labs.
AB: Good day Yuan, tell our readers a little bit about yourself.
YT: My name is Yuan Tung, and I am the Chief Technology Officer and Co-founder of ORO Labs. I’ve been working in the field of procurement since 2000 when I joined Ariba as Tech Lead for Ariba Network. Before starting ORO with Sudhir Bhojwani and Lalitha Rajagopalan in 2020, I served as Global Vice President of Operation Procurement at SAP where I led commercial strategy for Ariba Network and Procure-to-Pay solutions.
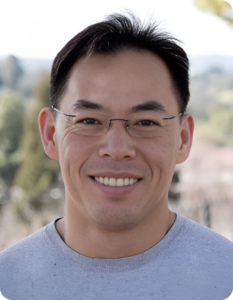
AB: Thank you. I think I know your answer but let me ask anyway … what are the biggest opportunities for AI to improve procurement efficiencies and productivity?
YT: Procurement is constantly running into data accuracy issues. The truth is that most of the data that is entered into procurement systems comes from untrained end users, which often makes data intake unreliable. AI can help with that.
There’s a common misconception that people primarily use procurement software to purchase items. In reality, most users aren’t buying anything through these systems. Instead, they enter information about purchases they’ve already decided on, often with a supplier quote in hand. Most users see this data entry as an unnecessary bureaucratic step and don’t prioritize accuracy, treating it as a mere handoff to procurement. That’s what creates data accuracy issues.
But we can’t really blame the users, because they don’t know how to “do it right” in the first place. If you present a user with 50,000 categories, for example, how can we possibly expect someone outside of procurement to select the right one? This is one area where AI comes into play, as it can help streamline the process, make it more intuitive, and decrease data accuracy issues by recommending the correct category code, buying channel, supplier, etc.
Another issue facing procurement that AI can help with is the user experience. From the end-user point of view, they often have very little visibility into the procurement process after they submit their request. This can lead to confusion and anxiety around what’s happening and how their request is progressing.
Really what you have here is the same problem from two points of view: Procurement is frustrated by poor data quality and the need for a lot of manual rework, and users are frustrated because they don’t know what’s going on. By using AI to help guide users through the procurement process, you actually address both issues.
Procurement is complex. The requirements for ordering a pen test in engineering differ significantly from what is necessary to order a t-shirt in marketing. By making the buying process more intuitive and transparent, you address user frustration and make sure that the data they provide is correct at the point of intake. Better usability leads to higher productivity.
AB: How are you ensuring the accuracy and reliability of customers’ AI models (AI data output)?
YT: Large language models (LLMs) can be used to check data quality and to tag data for use in building custom models. At ORO, we use LLMs to screen, correct, and enhance data, improving it to an acceptable level. While we experiment with ways to achieve 100% data quality, we understand that perfection isn’t realistic — or necessary — when working with massive data sets. Even involving humans in the process rarely leads to significant improvements, as human accuracy is often lower than we assume.
Second, especially for data that is mission-critical, we do a lot of checking against authoritative data sources. For example, we check for things like supplier names, email addresses, domain names, and bank account information against established registries to make sure they are correct.
AB: What are the leading AI subsets (e.g., machine learning, natural language processing, deep learning, etc.) with the biggest impact on procurement solutions? And why?
YT: When you look at the history of AI, the biggest jump in impact came as a result of the widespread availability of large amounts of training data. However, procurement data is often inaccessible due to its sensitivity, making it difficult to train AI effectively. This lack of available data has been the primary barrier to the widespread adoption of AI from within procurement — until now.
LLMs, trained with general knowledge available on the web, have significantly reduced the amount of training required to implement an AI model. In procurement, where transaction volumes are typically lower than in other domains, collecting sufficient training data has been a challenge. On top of that, procurement teams often lack the resources to tag data sets, a crucial step in training AI models. This has historically created two barriers to AI adoption in procurement: limited access to training data and the lack of personnel to prepare it.
With LLMs now trained on general knowledge, procurement departments can leverage them as a starting point, requiring minimal configuration to achieve results. The introduction of GenAI has effectively removed the burden of training AI models, making AI adoption far more accessible for procurement.
AB: How can you support procurement clients when an AI skills gap deficiency exists?
YT: Our job is to build a model specifically for procurement. Today, when we are hiring, we hire specific people with specialized skill sets to do certain jobs. We do not hire generalists to do work that requires significant expertise in a specific domain. The same is true when it comes to AI models. Going forward, we are going to start seeing more and more companies configuring their own proprietary models to perform specialized tasks. Just as they hire the right people for the job, they will build the right models to meet their unique needs.
Procurement professionals bring the most value through their deep industry expertise, which is their core competency. Managing procurement processes, however, is where vendors step in by building models designed to handle these tasks. Increasingly, vendors will develop specialized models tailored to their solutions, addressing specific procurement challenges. This allows procurement professionals to focus on their true value: advising the business on purchasing decisions and leveraging their industry expertise — not chasing down details or enforcing policies.
Our job as a vendor is to build models aligned to our core competency so that procurement leaders can focus on what they do best. It’s also our responsibility as an orchestration vendor to make it easy for organizations to embed their own models within processes should they choose to do so.
Orchestration exists to provide a bridge between people and systems, but also between models. Just like orchestration helps end users complete requests without forcing them to become experts in procurement, it helps procurement professionals use large language models without having to be experts in AI. Companies are increasingly building their own models in IT, and they will need vendors like us to hook them all together.
We are not looking to a future in which procurement professionals all of a sudden become citizen data scientists. Their job is to define processes. In the future, they will work more and more with IT to develop models to perform specific tasks. But even then, they will need to think about how various models work together as part of a process and work with vendors like ORO to connect models and processes so that they achieve well-defined outcomes.
For example, today, you don’t want AI to build a program for you from end to end. Instead, you have AI build functions that then need to be linked to work in concert.
LLMs are actually pretty easy to use and integrate. The real skills gap in procurement isn’t about understanding how AI works — it’s about knowing what AI can and can’t do. This knowledge is crucial for reimagining processes and identifying new applications and opportunities where AI can add value.
AB: How do you view your customers’ (and prospective customers’) AI attitudes?
YT: Everyone right now is looking at AI as more of a novelty. There’s a lot of excitement as people and organizations are starting to see the possibilities. In procurement specifically, however, people seem more focused on solving intake problems than forcing themselves to think about other implications. But as teams gain experience with technology and see it in action, they naturally start to uncover and pursue new opportunities.
AI has been in the background for a long time. It used to be fairly unapproachable, so people didn’t really think about how they could leverage the technology. GenAI is just bringing it to the foreground. Because of the growing popularity of large language models, people are becoming interested and more imaginative.
People are realizing that LLM outputs aren’t going to be 100% accurate all the time and are thinking about AI models much like they think about people. The question is more pragmatic. We’re moving away from asking “is it right?” and toward “is it usable?”. How people think about AI in that sense is increasingly more like how humans view working with other people than it is like how humans view working with machines. Just like they can’t expect the same input to yield the same output in human interactions, they can’t expect it from large language models. If there’s one area in which human AI literacy needs to improve, it’s getting used to interacting with powerful but fallible models in ways that require thoughtful and critical engagement.
AB: Where do you see AI solution offerings within procurement in five years?
YT: Everything comes back to cost. Running a prompt is still quite expensive. As prices come down, I believe that we’ll get to a point where everyone will build their own models. High-volume, labor-intensive work is going to be replaced by AI.
I don’t believe that in the next five years we will see AI being used in ways that are fundamentally different from how we use it today. Instead, we are simply going to see it being used more.
For example, think about the software industry. When the industry started, there weren’t a lot of software companies because of how expensive they were to set up. When the iPhone came along, we saw a huge explosion of app developers because they solved the selling problem. The selling of software became much cheaper. The same thing happened again with Amazon, which significantly decreased the cost of deploying software. Same thing with AI. As the cost of AI comes down, we’ll see an explosion of AI adoption.
The cost of training models will continue to be high, which means that the development of new models will likely continue to be something that only a few companies can do. But that’s not so different from the state of silicon fabrication today. There are only a handful of silicon foundries today, which everyone uses. Similarly, there will be just a few companies that are actively creating foundation models that the rest of the world uses and adapts to their purposes.
In procurement specifically, I don’t see a future for predictive intelligence in the same way as we see it in our personal shopping experiences. We don’t want procurement systems to predict what employees are going to want to buy and we don’t want to build systems that are trying to replace human judgment. Instead, I see a future in which AI is increasingly used to help guide humans as they attempt to navigate environments characterized by increasing complexity.
Why do we have lanes on the road? You need to set certain guidelines to help people coordinate their movements so that everyone ends up at their desired destination as safely and efficiently as possible. That’s what orchestration does: it establishes guidelines that help people move around an organization so they can get work done safely and efficiently.
As we look to the next five years, procurement’s job is increasingly going to be that of a roadbuilder and navigator, helping to establish the infrastructure that helps people to move so that employees can easily follow the best road to get them from point A to B.
AB: I appreciate your time today!
YT: Thank you.